« To fully understand the extent of the disruption brought by generative IA we can make a type of analogy and remind that it is an inflexion point that is comparable to what happened 20 years ago with cloud computing on the global IT landscape. The extent of this disruption explains why generative IA become one of the key areas of transformations and innovations for large companies like financial companies… » .
Christophe SORRÉ, CTO for Financial Services & Consumer Industry at IBM France.
In this episode, tune in to Christophe SORRÉ as he unravels how generative AI is shaking up the banking and financial industry. Because if generative AI is experiencing rapid growth and attracting increasing interest both in the research domain and in industry, it also raises significant challenges in terms of ethics, society, and regulations… This issue has fueled the various questions that punctuate this interview, including:
- What does generative AI bring to the table that classic AI doesn’t, and how does it set itself apart?
- What are the most striking or useful initial use cases you’ve observed in the financial domain?
- Specifically, what kinds of tasks will change in the banking and financial industry?
- How do we prepare for transformations when generative AI is constantly evolving?
- What will be the human acceptance level of interacting with robots?
- How do we prevent “hallucinations”?
- Does it make sense to develop AI given the current ecological footprint?
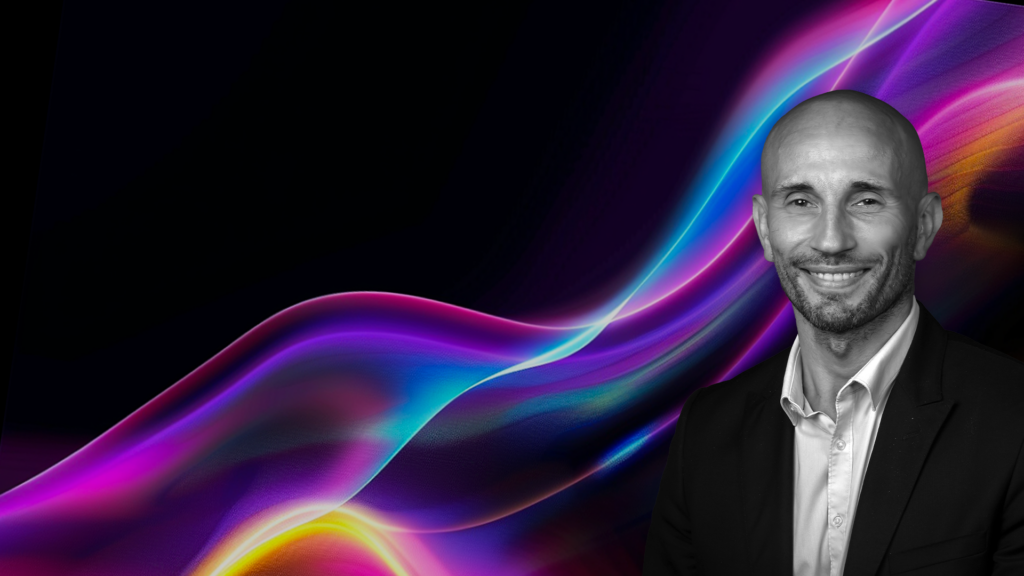
Transcript of the podcast
Generative AI and its distinction from traditional AI+–
Caroline: Welcome to FinTrends, a podcast series focusing on the latest trends and news in the financial sector, brought to you by Sopra Banking Software. We’re joined by experts to dive into the industry’s most pressing topics. I’m Caroline Beguin, and today, I have the pleasure of speaking with Christophe Sorré about the transformative impact of generative AI in the banking and financial sectors. Welcome, Christophe.
Christophe: Thank you, Caroline. It’s great to be here.
Caroline: Christophe serves as the CTO for financial services and consumer industries at IBM France, spearheading the technical strategy within these domains. Additionally, he’s a valued member of the IBM Academy of Technology. Positioned at the forefront of IBM’s innovation, Christophe is deeply immersed in the advancements of generative AI, a breakthrough promising to redefine business operations. The question at hand is whether this marks a threat or an opportunity. To set the stage, Christophe, could you enlighten us on generative AI and its distinction from traditional AI?
Christophe: Absolutely, Caroline. Grasping the full impact of generative AI requires drawing parallels to a pivotal moment in history – the advent of cloud computing two decades ago, which significantly reshaped the IT landscape. This comparison underscores the potential of generative AI as a cornerstone of transformation and innovation for corporations, especially within the financial services sector.
Traditional AI frameworks, including deep and machine learning, are tailored to specific tasks through model training. Conversely, generative AI operates on foundation models like large language models, constructed from vast arrays of diverse, unlabeled data supplemented by proprietary business insights. This self-supervised learning approach leverages both external and company-specific data, fine-tuned to support a broad spectrum of applications from conversational aids and document synthesis to content generation and coding support.
What sets generative AI apart is its ability to produce novel content – be it text, images, or audio – in response to queries or prompts. This concept of ‘prompt engineering’ represents just one facet of leveraging generative AI, underscoring the importance of its integration within core IT and business processes to enhance efficiency and service offerings. Generative AI stands ready to address inquiries from management or interact with system-generated requests, showcasing its versatility and potential to revolutionize the industry.
Caroline: Generative AI stands as a formidable catalyst for innovation. Could you share some of the most impactful or practical applications you’ve observed within the finance sector?
Christophe: That’s an insightful query, Caroline. While the potential applications are vast, certain high-value use cases stand out for banks and insurance companies, our clientele. Notably, advisor assistance has seen remarkable advancements; advisors are now equipped with a comprehensive, 360-degree customer view, enabling ultra-personalized interactions and tailored service or product recommendations that resonate with individual customer profiles.
In the realm of financial service portfolio management, generative AI empowers advisors with customized recommendations, enhancing customer trust and driving growth for the institution. Furthermore, it’s reshaping digital banking, our daily go-to, by fostering new, trust-enhancing interaction channels, thereby elevating consumer satisfaction through quicker, more precise, and interpretable responses.
Risk management is another critical area; generative AI models trained on historical data can assess new prospects in real-time with unparalleled accuracy, even incorporating volatile factors like the current fluctuating interest rates into their evaluations. This capability extends to anticipating risks and default probabilities, offering a broadened scope beyond credit scoring to encompass systemic risk management in commercial banking. Here, the technology aids in simulating potential crises and identifying financial system vulnerabilities, allowing for proactive risk mitigation.
Regulatory compliance, too, benefits immensely from generative AI. It simplifies and automates the complex processes of aligning business practices with regulations such as NIST, PCI DSS, DSP2, and the forthcoming Digital Operational Resilience Act, due in January 2025. This not only forecasts compliance needs but also significantly reduces the manual effort involved in validating control postures and governance.
Lastly, in fraud detection, generative AI’s ability to proactively identify intricate or elusive patterns—beyond human analytical capacity—stands out. This enhances operational efficiency, cost savings, and satisfaction among customers and employees alike, showcasing the vast, untapped potential generative AI holds for the financial industry.
AI reaching its zenith within the financial sector+–
Caroline: Looking ahead, where do you see generative AI reaching its zenith within the financial sector?
Christophe: This ties back to our discussion on key applications. Foremost, the reinvention of banking experiences for advisors and customers through instantaneous, personalized interactions is paramount. This not only improves conversion rates for new products and services but also fosters customer loyalty.
Another critical domain is application modernization, where generative AI injects a wealth of innovative ideas for rapid advancement. Despite the reliability of legacy core banking systems, often running on stable yet dated mainframes, the dwindling pool of resources for managing and updating these systems poses a challenge. While it’s unrealistic to expect generative AI to instantly translate legacy code like COBOL into modern languages with a mere click, it facilitates a targeted approach, enabling parts of the code to be translated and integrated seamlessly with the remaining legacy systems.
Caroline: The implications of generative AI on job evolution remain somewhat uncertain, yet it’s clear that its integration will alter professional practices. Can you shed light on how specific tasks might evolve within the banking and financial industry as a result?
Christophe: Caroline, your question touches on a spectrum of sentiments surrounding generative AI, from optimism and curiosity to skepticism and concern. I’d like to address this through various lenses. For instance, consider a bank advisor evaluating consumer or real estate loans. Generative AI could enhance reliability in scoring systems, providing clear justifications for decisions in a high-interest rate environment, thus bolstering the advisor’s confidence in their daily operations, including the overwhelming influx of client emails. Many of these are routine inquiries that generative AI can resolve autonomously, freeing advisors to focus on higher-value tasks, with clients feeling personally attended to by their advisor, not a machine.
For marketing teams, generative AI offers tailored product recommendations that resonate with the nuanced needs of diverse customer segments. This tool is invaluable in aligning offerings with consumer expectations. Developers, too, stand to benefit by pivoting from coding to optimizing application architectures, thus enhancing their skills and contributing more significantly. Similarly, traders can leverage real-time data augmentation in their advisory systems to better assess transaction risks.
In essence, generative AI has the potential to support or streamline nearly all human-centric tasks, paving the way for more efficient and effective operations across the board.
How can organizations prepare for the ongoing transformations generative AI brings?+–
Caroline: Given the rapid evolution of generative AI, how can organizations prepare for the ongoing transformations it brings?
Christophe: The crux of the challenge for our clients isn’t whether to adopt generative AI, but how to strategically and governance-wise navigate its integration. Many companies have been engaging with AI, including machine learning and deep learning, for over a decade, gradually incorporating these technologies into their operations. However, the transformative capacity of generative AI, potentially boosting turnover by up to 5%, positions it as a pivotal change agent.
The path to integration involves two key strategies. Firstly, adopting use cases by proactively identifying and categorizing potential applications based on value contribution and implementation costs. Secondly, embedding generative AI within all transformation initiatives, leveraging the technology where it offers the most strategic advantage.
The adoption journey can be segmented into four stages: acculturation, ideation (identifying and conceptualizing use cases), piloting through proofs of concept and minimum viable products, and, finally, change management to adapt operational models accordingly. To navigate this transition successfully and maintain a competitive edge, organizations must establish a coherent adoption framework, underpinned by a robust data and AI architectural foundation.
Is there a risk that generative AI might make this technology obsolete?+–
Caroline: Considering the collaboration between Sopra Steria and IBM in developing a conversational assistant, is there a risk that generative AI might make this technology obsolete?
Christophe: Caroline, you’re alluding to the collaborative effort on Live Intelligence, a notable asset from Sopra Steria and IBM. It’s a pertinent question. However, generative AI is not a threat to this innovation; rather, it’s set to enhance and expand the capabilities of the conversational assistant integral to Live Intelligence. Currently, our teams from Sopra Steria and IBM are actively evolving the Watson conversational assistant into a more advanced version powered by generative AI technologies like Watsonx. This evolution is expected to yield more comprehensive and concise responses, drawing from a broader spectrum of organizational data, all refined by a large language model trained for precision, thereby improving the accuracy, traceability, and overall performance of the digital assistant.
Caroline: When can we anticipate this enhanced conversational agility to be implemented across all banking touchpoints?
Christophe: I might temper expectations slightly by saying it’s contingent. The timeline largely depends on our clients’ deployment strategies and the current state of their IT infrastructure, especially concerning data architecture. The crux of the matter often ties back to data when discussing AI’s capabilities. While the technology to support advanced multi-channel conversational assistants is ready, the real challenge lies in harmonizing access to internal and external data sources necessary to feed generative AI models.
Many banks have already embarked on extensive big data initiatives to bolster data science and business intelligence functions. However, transitioning from traditional data lake architectures to more generative AI-friendly ‘lakehouse’ models, which incorporate enhanced governance and metadata layers, is crucial for swift and successful adoption.
With this in mind, and considering the prerequisites, a bank with an existing B2B or B2C conversational assistant framework could feasibly achieve greater interoperability across branches and business units within 6 to 12 months through generative AI integration. Yet, it’s important to approach these projections with caution, as project timelines can vary based on a multitude of factors, necessitating a degree of flexibility in planning.
Foreseeing the future of B2B interactions with generative AI+–
Caroline: With the advent of generative AI, how do you foresee the future of B2B interactions? And how ready are people to engage with an increasingly automated, albeit sophisticated, digital landscape?
Christophe: Caroline, it’s somewhat premature to fully predict the impact on B2B dynamics. Currently, generative AI is met with a mix of excitement and apprehension, varying by the audience. Business leaders and CXOs, for instance, view it as a pivotal topic, driving enthusiastic discussions and numerous exploratory projects to gauge its potential. On the other hand, those in roles potentially streamlined by automation, like developers and legal assistants, express some concerns about their future responsibilities.
It’s crucial for organizations like Sopra Steria and IBM to educate various stakeholders about generative AI’s role—not as a replacement, but as a tool to augment human capabilities. The aim is to create a seamless interaction with digital assistants, making it indistinguishable whether one is conversing with a machine or a human. If the digital interaction is accurate, swift, and tailored, the nature of the respondent becomes less relevant. Ultimately, the challenge for companies isn’t about job displacement but staying competitive by adopting generative AI effectively.
Caroline: In a sector as critical as finance and banking, can we afford to rely on tools like generative AI that may err?
Christophe: The concern is valid but needs context. In financial services, crucial operations like payments and loan servicing remain under strict human oversight, a practice unchanged by the advent of AI. Even with AI’s recommendations, decisions like credit scoring rest with human professionals. While generative AI might produce inaccuracies or ‘hallucinations,’ these outputs don’t directly feed into core banking systems without thorough human validation.
The industry’s focus is on ensuring responsible, transparent, and explainable AI practices to minimize errors and maintain traceability throughout the AI lifecycle. Regulatory frameworks, like the AI Act in Europe, are evolving to govern AI’s use, emphasizing systems that pose significant risks while promoting enterprise-level responsibility for lower-risk applications. Financial institutions will need to demonstrate their commitment to these principles, ensuring human oversight, risk mitigation, high-quality data, and secure, robust AI implementations.
Generative AI and ecological print+–
Caroline: Considering the resource-intensive nature of generative AI, how do we reconcile its development with the pressing need to reduce our ecological footprint?
Christophe: Caroline, your question touches on a critical balance between technological advancement and environmental sustainability. The development of large foundational models for generative AI indeed requires significant computational resources. However, advancements in automation and optimization offer a counterbalance by potentially reducing the environmental impact. The challenge lies in finding equilibrium: selecting more compact, function-specific models that consume fewer resources while still delivering substantial energy savings in their application domain. Despite the current focus on exploring AI’s capabilities, the sustainability aspect is becoming increasingly crucial in the final selection of technologies. At IBM, we’re committed to providing cloud-native infrastructure optimized for AI, boasting significant computational power while promoting efficient resource usage. This approach ensures that clients can scale their AI initiatives responsibly, aligning with sustainability goals.
How do you measure the scope and scale of a generative AI project?+–
Caroline: How do you measure the scope and scale of a generative AI project?
Christophe: The scope of a generative AI initiative hinges on the chosen use case. For instance, enhancing a conversational assistant in retail banking might span a few weeks to a couple of months, involving a modest team. Conversely, more complex IT-related projects, like incident summarization, could extend over several months for a large banking client, reflecting the project’s intricacy and integration requirements with existing IT systems. The project’s duration and complexity primarily depend on the number of integration points with the client’s data architecture. Some clients may pursue a comprehensive generative AI platform to centralize various applications, which would extend the project timeline. Like traditional AI, generative AI leverages service-based solutions with adaptable models, distinct from the extensive commitments seen in back-office transformations or multi-channel integrations.
Caroline: Thank you for the insights, Christophe.
Christophe: It was my pleasure, Caroline. Thank you.
Caroline: Goodbye.